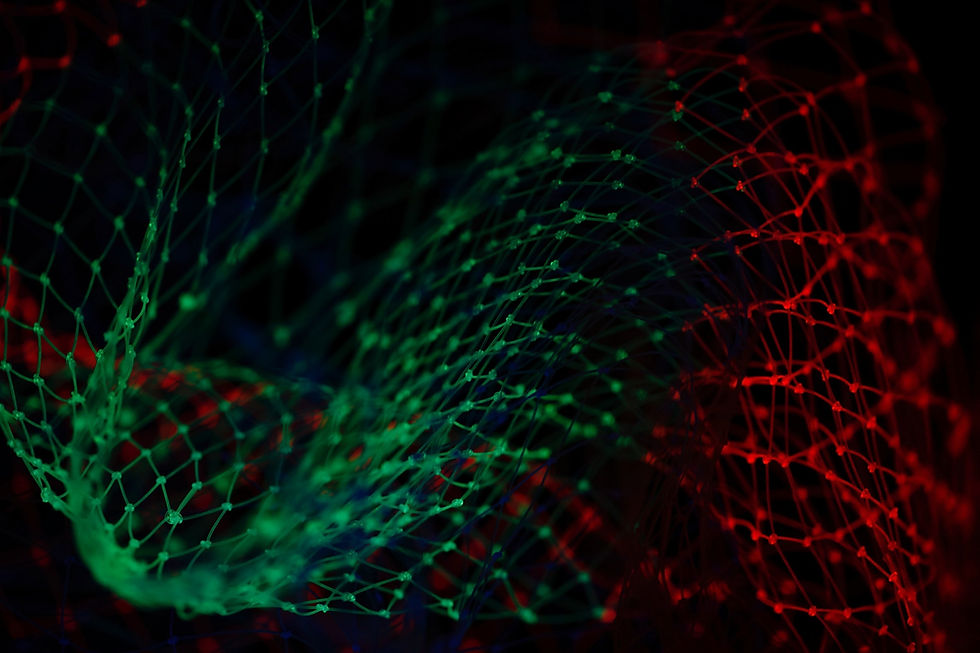
This is a topic that admittedly confuses me too, even though I'm an Actuary. I'm presenting my thoughts here to shed some light both for those interested and for myself.
Disclaimer - I'm an Actuary, so please forgive me for my bias and limited knowledge of the Data science field.
Actuarial science and Data science are adjacent professions which share their foundations in data analytics. Both use analytical methods to understand the past using data, build models to understand future possibilities and provide insights for decision making.
The Actuarial profession places a very high importance on professionalism and standardised education and credentials that require passing rigorous examinations and assessments. Even though there are a number of different international actuarial bodies (SOA, CAS, IFOA, IAA, etc), their syllabus tends to be fairly synchronised to reflect the outlook of the profession.
The curriculum involves key mathematical and statistical concepts, insurance concepts, the Actuarial control cycle approach for problem solving, and specialist material in various insurance, banking, finance, risk management or other chosen fields.
The Data science profession is a liberal one with many different certification programs, various pathways and requires each individual to chart their own path for their education, credentials and professionalism. This does result in a large field of data science practitioners.
Many data science professionals get a Masters degree or PhD to demonstrate their superior competence in the field.
Actuarial organisations have started offering credentials and certifications for data scientists too, adding in the professionalism and control cycle elements.
The Actuarial profession has tended to focus heavily on the insurance profession, but thankfully has began to expand its horizons. Most practitioners are still within the insurance, reinsurance and consulting (mainly consulting for insurance) fields, with some in the banking and other areas.
The Data science profession has no such limits. Data practitioners are everywhere where data is available. They are in the insurance field too and in e-commerce, travel, telecommunications, banks, consulting, and in any field where the business needs to organise and analyse data to derive insights. This means that there are many more data analysis and data science roles available compared to actuarial roles.
The tools used in both professions can be fairly similar with both using Excel, VBA, SQL, R, Python and others. But they also diverge where the actuarial profession has specialised software such as Prophet, AXIS and the like, whereas the Data science profession uses many others for unstructured data as well. It is fair to say that most Data scientists go further than most actuaries in using statistical programs.
Actuaries do many things beyond data analysis, including product design, risk management, strategy, investments and others. By broadening their scope actuaries take their careers further as well.
The career pathway for Actuaries can take them to the very top of insurance, reinsurance and consulting organisations, with many helming top posts such as CEO, Managing Director, CFO, Chief Actuary and others.
Data scientists tend to be specialists in their areas and can become the Head of Analytics. Some may broaden their scope by taking on other roles as well and may pave pathways to the top of their organisations too.
An analytical undergraduate degree is important for both fields. Although actuarial science degrees have become popular in recent times, they aren't mandatory and one can certainly be an Actuary without one.
Data scientists can certainly also become Actuaries too by getting the actuarial credentials through the professional examinations and assessments.
Actuarial candidates who don't opt to proceed further with their actuarial credentials can apply their skill sets in the data science field too.
I hope this sheds some light for you to understand these fields better, and helps you navigate your career path as well.
Comentários